Data Quality is A Top Priority
One of the biggest challenges creating and nurturing a data driven decision making cultures is data quality. Every organization has data in different systems. As a result of system defaults or historical decisions there is never one consistent definition across every system. This means, when IT or Business Users begin merging data from on-premises or cloud systems they often get stuck in data quality and inconsistency issues from different systems. Data cleansing, the process of identifying and rectifying quality issues such as errors or inconsistencies in datasets, is a critical step in ensuring the accuracy and reliability of business insights
For example, how often do you see state or country names entered differently across systems. One system may use two letter state codes while another system will use full names. Let's take the state of California as an example, our human brains will recognize CA and California are the same. But to a data query engine, these are two distinct items in the state field. Other common data quality issues across systems are found in customer name fields, middle initials, phone numbers, countries, text case, spelling errors, and more.
Traditional tools like Informatica, IBM, Oracle, SAP, Tibco, and others are used by IT experts who can apply robust and often complex functions to clean and normalize data from different systems as part of a bigger data extraction, transformation, and load process into a target data warehouse. But this is where and when business users get frustrated. Why does data cleaning need to be so hard and controlled by only a few?
Can there be an easier way?
Luckily new tools like eyko have introduced a simplified workflow for business users to clean data as they are connecting to and merging information from multiple sources. eyko's users leverage the inbuilt smarts (machine learning) to automate the detection and correction of errors in datasets. These algorithms can analyze vast amounts of data rapidly, identifying patterns, anomalies, and inconsistencies that may go unnoticed to the human eye.
One of the key advantages of eyko's data cleansing is its ability to handle large and complex datasets with ease. Traditional methods of data cleansing often struggle to scale effectively, particularly when dealing with complex ERP systems and new SaaS applications. The inbuilt AI algorithms, on the other hand, excel at processing vast volumes of data efficiently, enabling organizations to cleanse their datasets quickly and effectively, regardless of size or complexity.
Here is an example of everyday data items, like phone numbers and e-mail addresses, that can be standardized in seconds using eyko.
Here is an example of before and after eyko's smart data cleansing is applied to data.
To learn more about how eyko can make data cleaning easier for everyone click here to watch the demo.
Share this
You May Also Like
These Related Stories
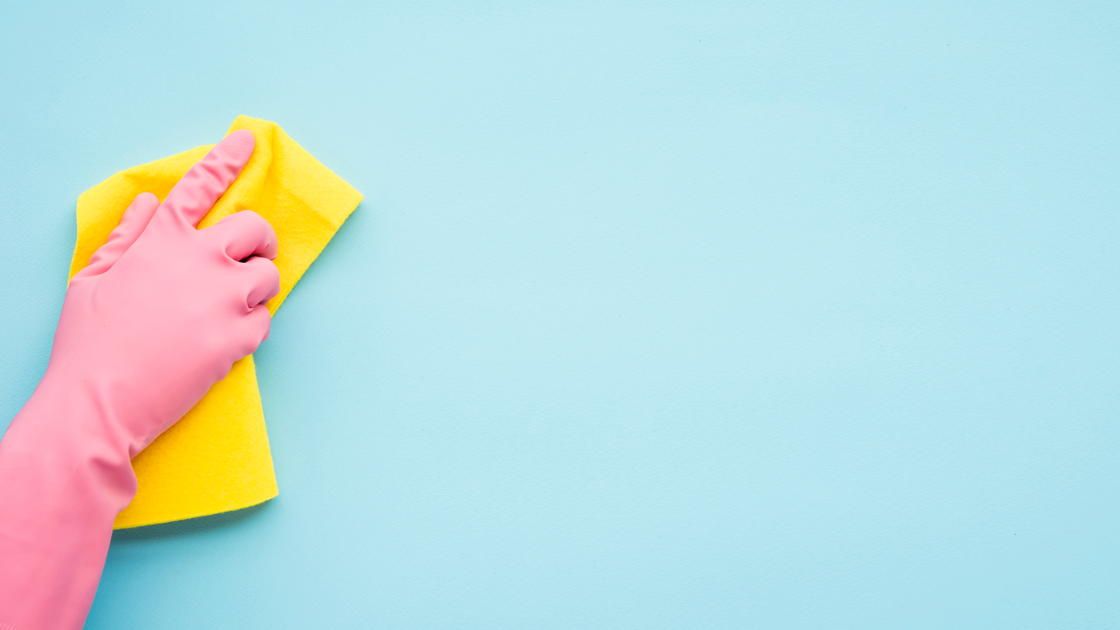
Clean data – Is the juice worth the squeeze?

If you like Microsoft Fabric, You’ll Love eyko
